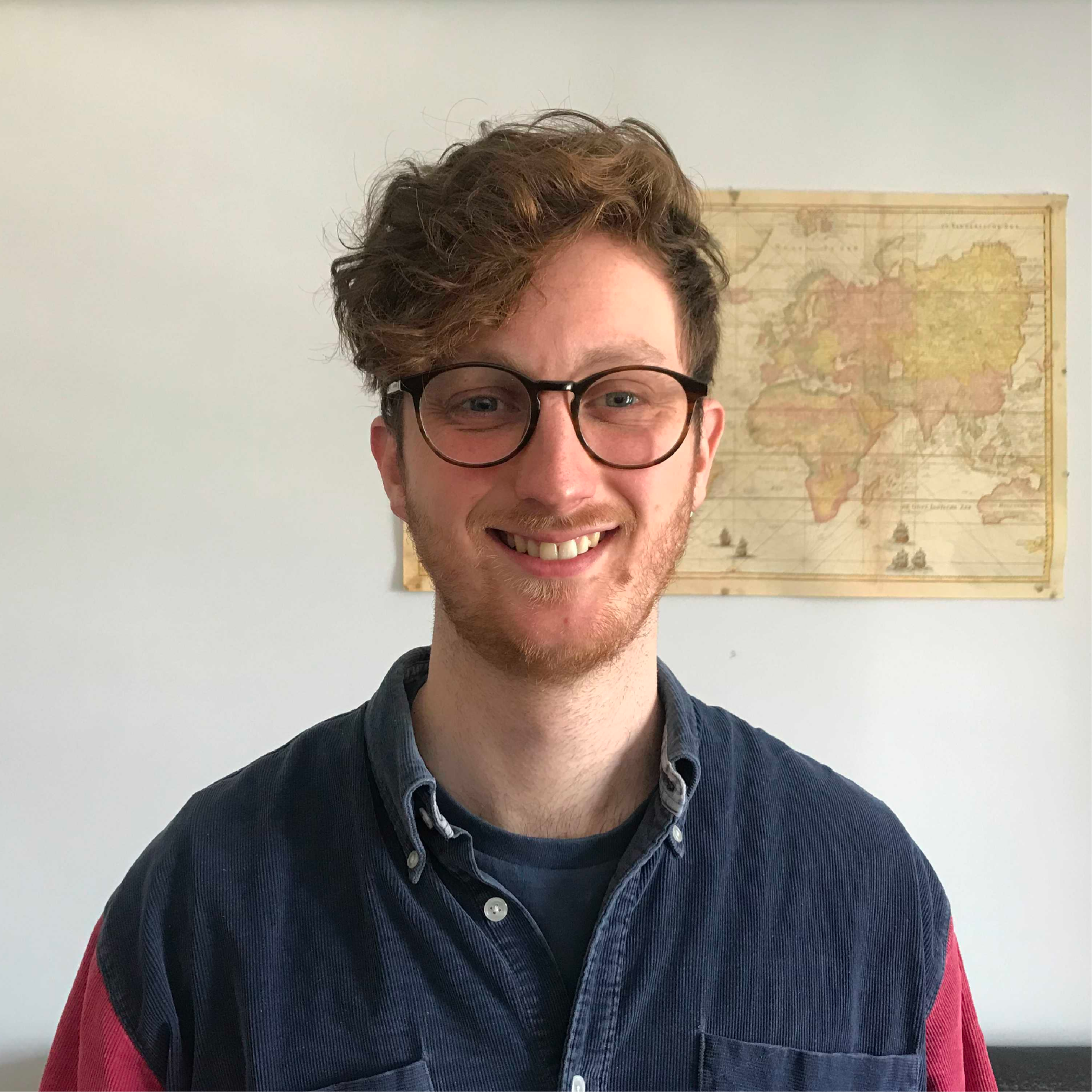
How to Study Brand Conversations with Advanced Text Analysis?
Never has social media been as important as it is today. The average time spent on social media is 145 minutes, or 2 hours and 25 minutes every day. The correct post can boost brand image or ruin it, and influencers can now be more famous than soap opera stars. It is no secret that the ability to capture this data allows companies a significant marketing advantage.
"It turns out that with Twitter data alone, we can go quite some way into figuring out someone's personality."
Twitter holds a wealth of qualitative data and is often used by researchers because of the ease with which we can collect tweets using Twitter's API. Tweet authors are likely to be opinionated, leave reviews and express happiness or displeasure in the face of products, services and companies at large. So how can we collect this data and carry out Twitter analytics in order to create useful insights to understand our market through the thoughts and feelings of the customers that drive it?
Scraping Data from Twitter
Text analysis always starts with data. Here, my first move was to scrape data from Twitter and use it to clean, prepare and construct a dataset of context-specific tweets.
To do this, I used the scraping tool - Tractor - built by the team of data scientists and engineers at Graphext. I wanted to learn more about the conversation topics surrounding my bank - Lloyd's Bank. To collect context-specific tweets here, I used the Twitter query: "Lloyd's Bank" OR "#Lloydsbank" and set the scraper to scrape all reply tweets and quotes about the bank.
I collected all tweets about Lloyd's bank from the past year to monitor and analyze the full conversation taking place on Twitter about the company.
Aware of the scale of the company and wanting to work with a manageable dataset size, I set Tractor to scrape tweets posted between the first of June 2020 and the 1st of June 2021. This twitter query gave me 3610 rows of data, which Tractor transformed into a usable CSV file that I could drag and drop straight into Graphext.
Setting Intentions for a Text Analysis of Twitter Data
So now that I had the dataset ready, I started to think about how to approach the analysis. Twitter data has a diverse range of use cases; competitor analysis, Twitter network analysis or conversation monitoring to name a few. It is worth bearing in mind that there are a number of purpose-built social media and Twitter analytics tools out there to carry out these types of data analytics projects.
But since Graphext can deploy advanced NLP algorithms and automatically clusters tweets in a network visualisation - I'll be building analysis with Graphext's Social Media - Topics analysis type. This way, I don't have to code with Python or R and have access to out-of-the-box data enrichments and machine learning models like sentiment analysis trained specifically on Twitter data.
Starting social media analytics projects from scratch used to be laborious - involving moving back and forth between marketing and data teams - but no-code tools empower people that don't know how to program to conduct the analysis themselves. In less than five minutes I had the graph below.
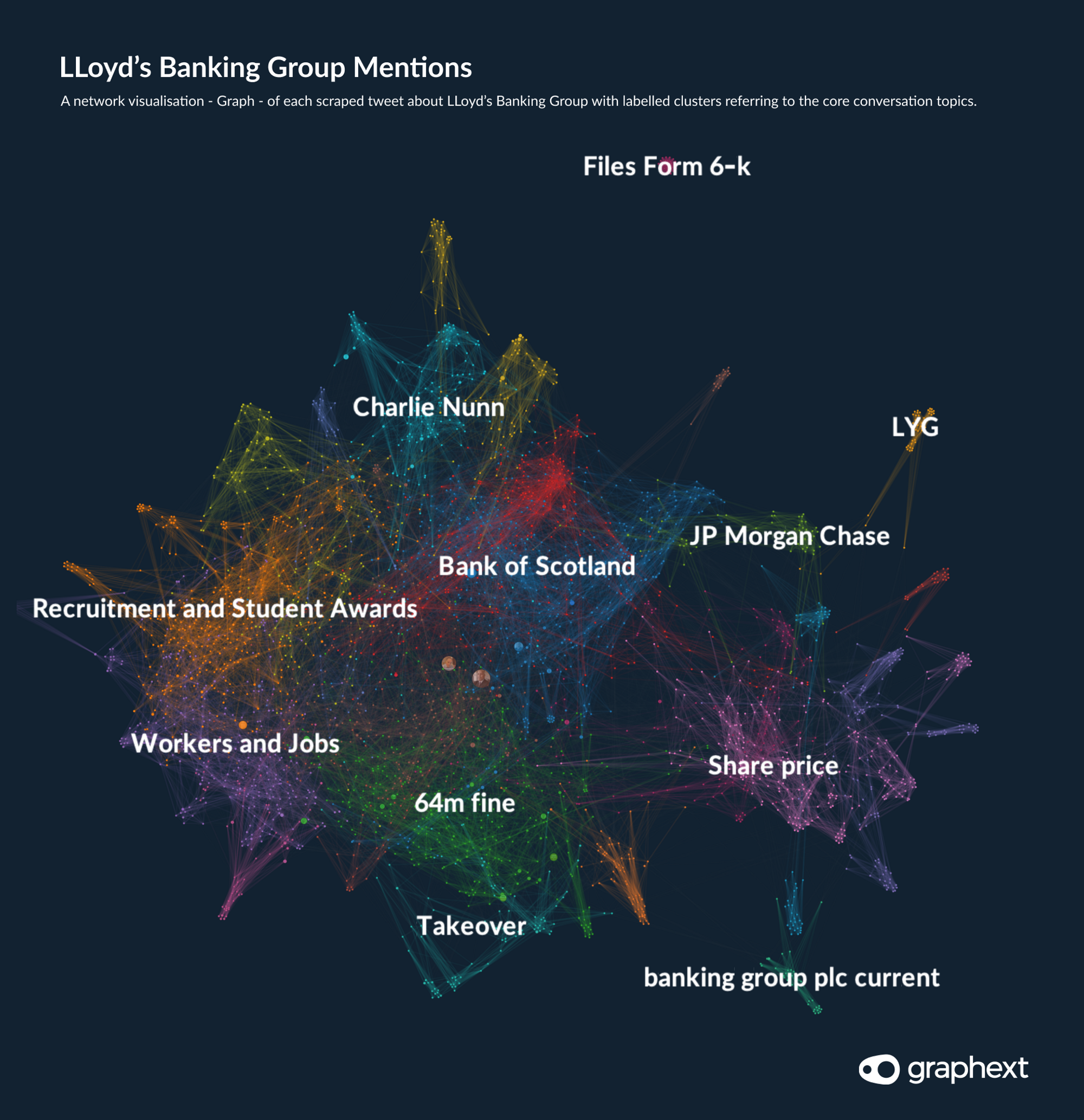
Graph: Clusters of tweets revealing the topics of conversations about Lloyds Banking Group.
After taking in the network of tweets that Graphext created, the first thing I did was try to have an idea of why our clustering analysis grouped tweets together; what kind of tweets are strongly associated with one another to form the conversation topics represented in our clusters?
Analyzing Conversations on Twitter: A Cluster of Tweets about Competitors & Subsidiary Groups
In this particular study, the largest cluster was focused around the banking group itself, its rivals and its subsidiaries. Our algorithm had grouped tweets together here because they often referred to the same businesses, banks or entities. I found that the topics automatically identified were not offering any meaningful insights.
To rectify this I created an automatic segmentation that split the cluster into 9 smaller sub-clusters. This way, I was able to inspect the topics surrounding the bank's competitors in more depth and found that the topic that was most tweeted about in the cluster regarded an alleged fraud from a subsidiary of Lloyd's HBOS.
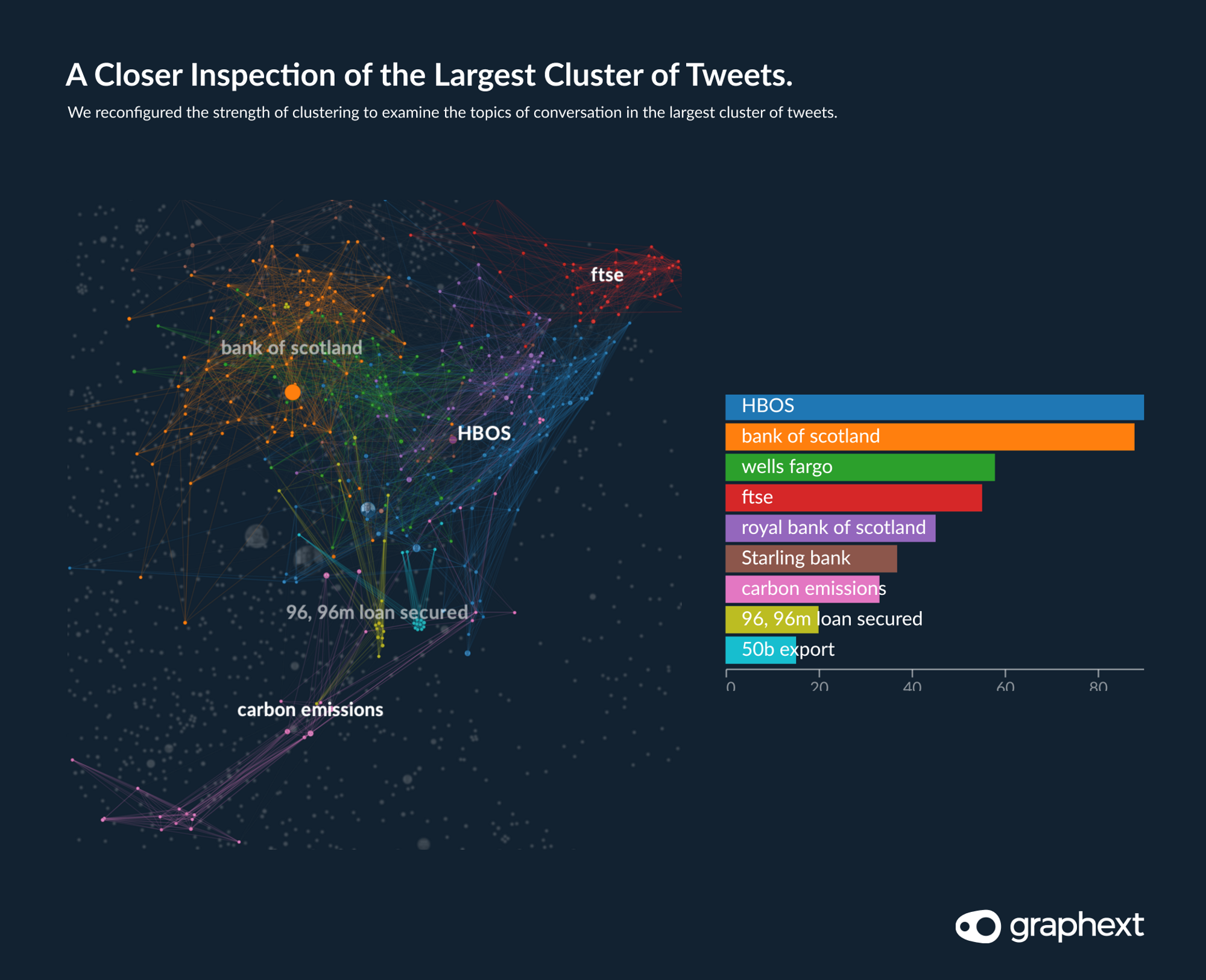
Graph: We auto-segmented tweets in our largest cluster to inspect the topics in more detail.
Analyzing Conversations on Twitter: Reactions to Business News
In this particular dataset, I found a number of interesting clusters that would be valuable to analyse. But a cluster of tweets that stood out to me immediately regarded the conversation about a merger with the fintech company Form3. Piquing my interest, I decided to analyse this with Graphext's Trends panel in order to investigate how engagement with the merger evolved over time.
The time-series analysis of this specific Twitter conversation showed that the majority of the discussion was held in July (25%) as the news broke. Afterwards, there were short bursts of interest throughout the year.
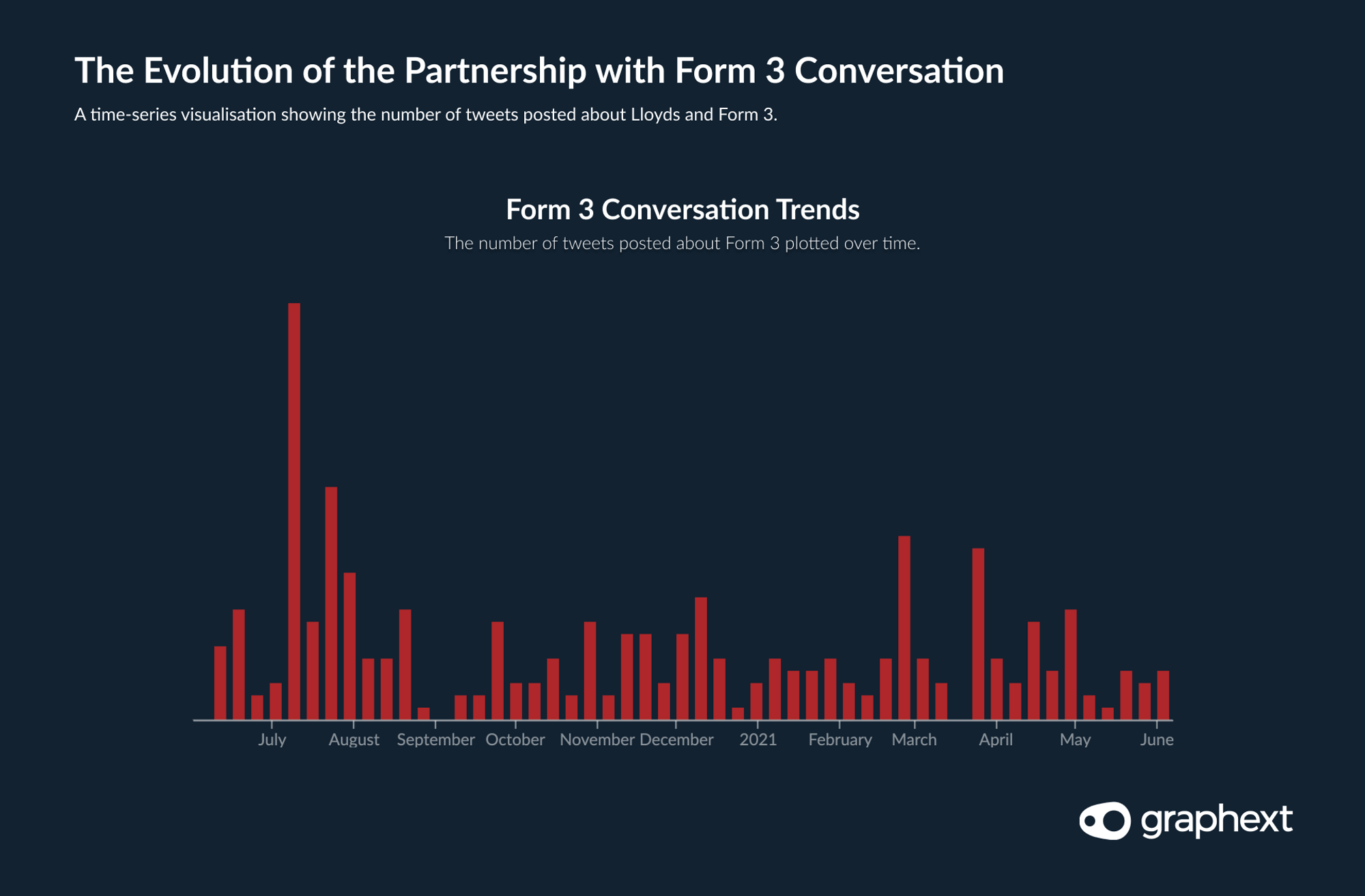
Trends: How the conversation about the partnership between Lloyds and Form3 evolved over time.
Analyzing Conversations on Twitter: How People Feel About Job Cuts
One of the benefits of clustering text data is the ability to spot patterns that would be lost in an excel sheet or a pivot table. For example, only 1% of the dataset refers to tweets mentioning job cuts. However, by filtering these tweets using the interactive network visualisation, we can start to analyse their features & content immediately. We were able to see that job cuts were only mentioned 51 times throughout the year with the majority of these tweets posted in November.
This should be seen as a huge success for PR damage control - since 1000 redundancies could have caused much more of a stir.
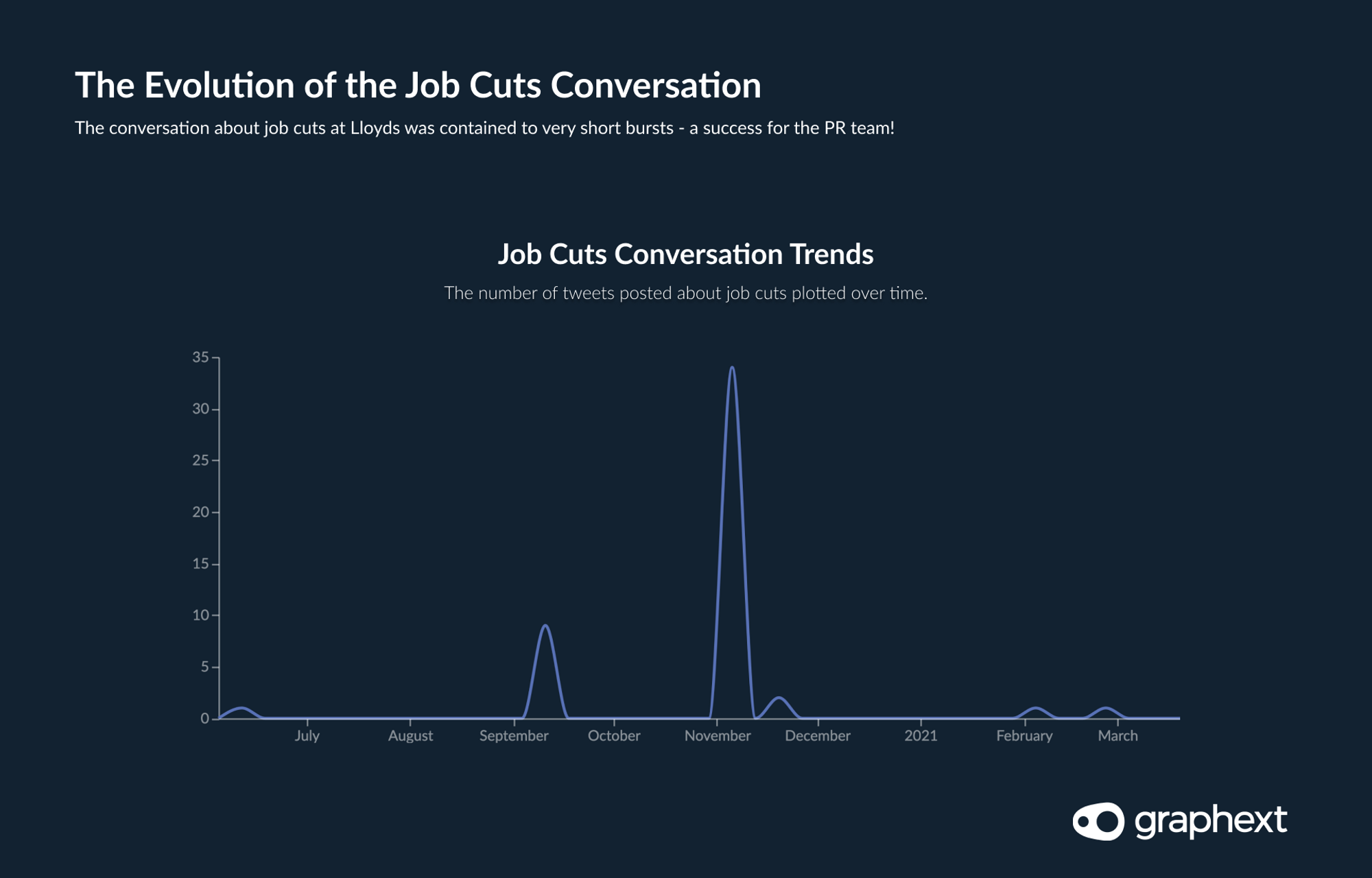
Trends: How the conversation about job cuts at Lloyds evolved over time.
Analyzing Conversations on Twitter: Sentiment Analysis
Sentiment analysis is a powerful NLP technique used to measure the positive or negative sentiment contained in the way that people speak or write. Graphext offers a number of integrated sentiment analysis models that are trained on context-specific social media data and are ready to deploy quickly and easily.
One of these is a Hugging Face model for sentiment analysis, built by data scientists at Cardiff University, and which has been integrated into Graphext. Using this sentiment analysis machine learning model, we can easily see which tweets were negative, neutral or positive and even identify how conversation sentiment evolves over time.
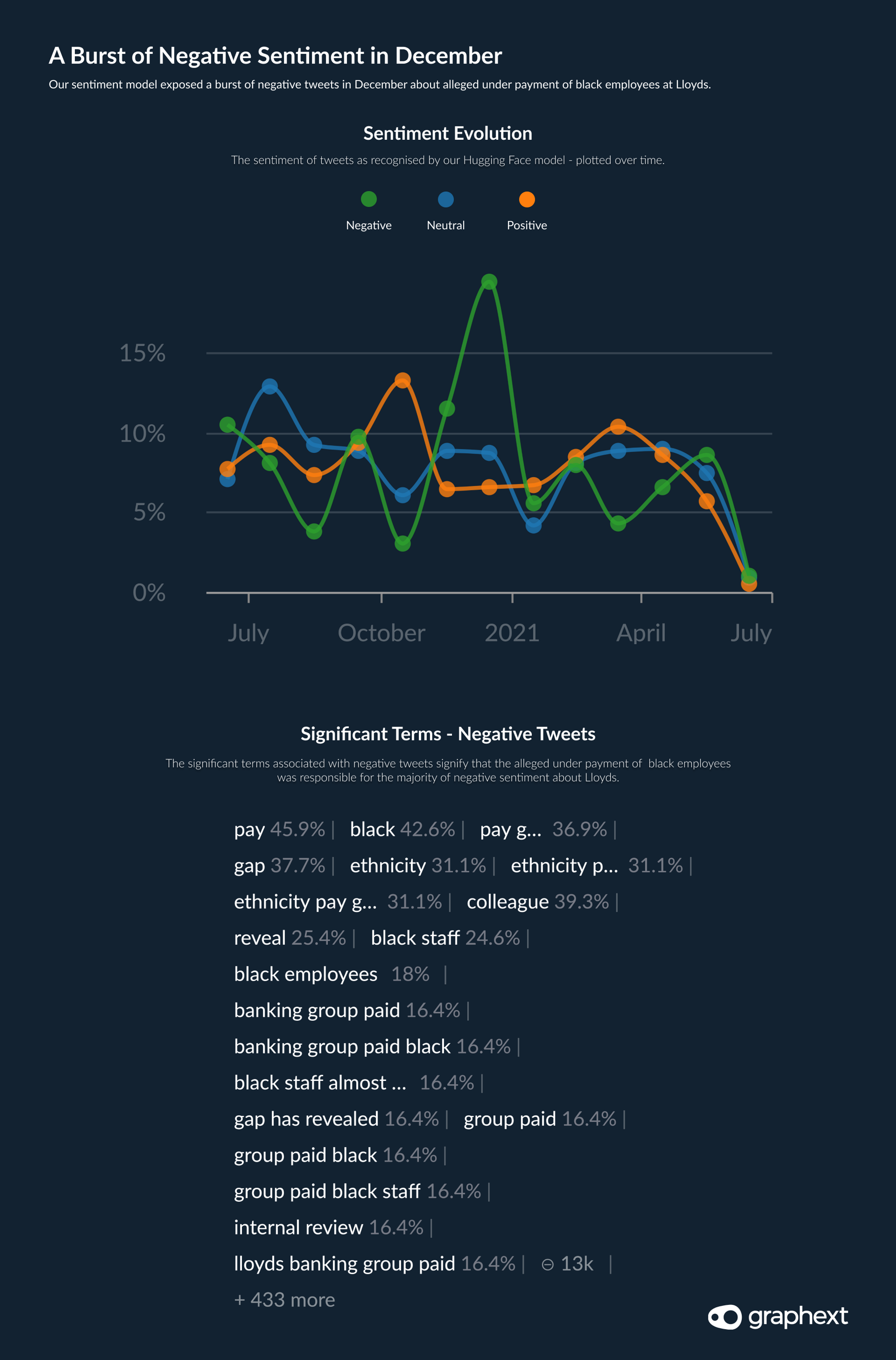
Compare & Significant Terms: Negative tweets about Lloyds burst through in December because of alleged under payment of black employees.
Analysing this in the Graphext's Compare panel, we can see that the majority of tweets are neutral or positive with only 17% of tweets being negative. However, I spotted a spike of negative tweets in December, 2020 motivating me to dive deeper into this segment of tweets. Closer inspection revealed that this particular segment of negative tweets about Lloyd's bank was referring to the issue of pay discrepancy between black staff and their peers.
Analyzing Conversations on Twitter: Finding Influencers
Another area that is receiving more and more traction within social media analytics, is the importance of finding influencers.
When we think of influencers many people go straight to thinking about young, beautiful people sponsoring and selling products. But influencers can also have negative effects on PR. With a company like Lloyd's, whose product is not so tangible, using social media network analysis to find influencers driving negative messages about the brand is an essential way of performing a root cause analysis to stop negative PR.
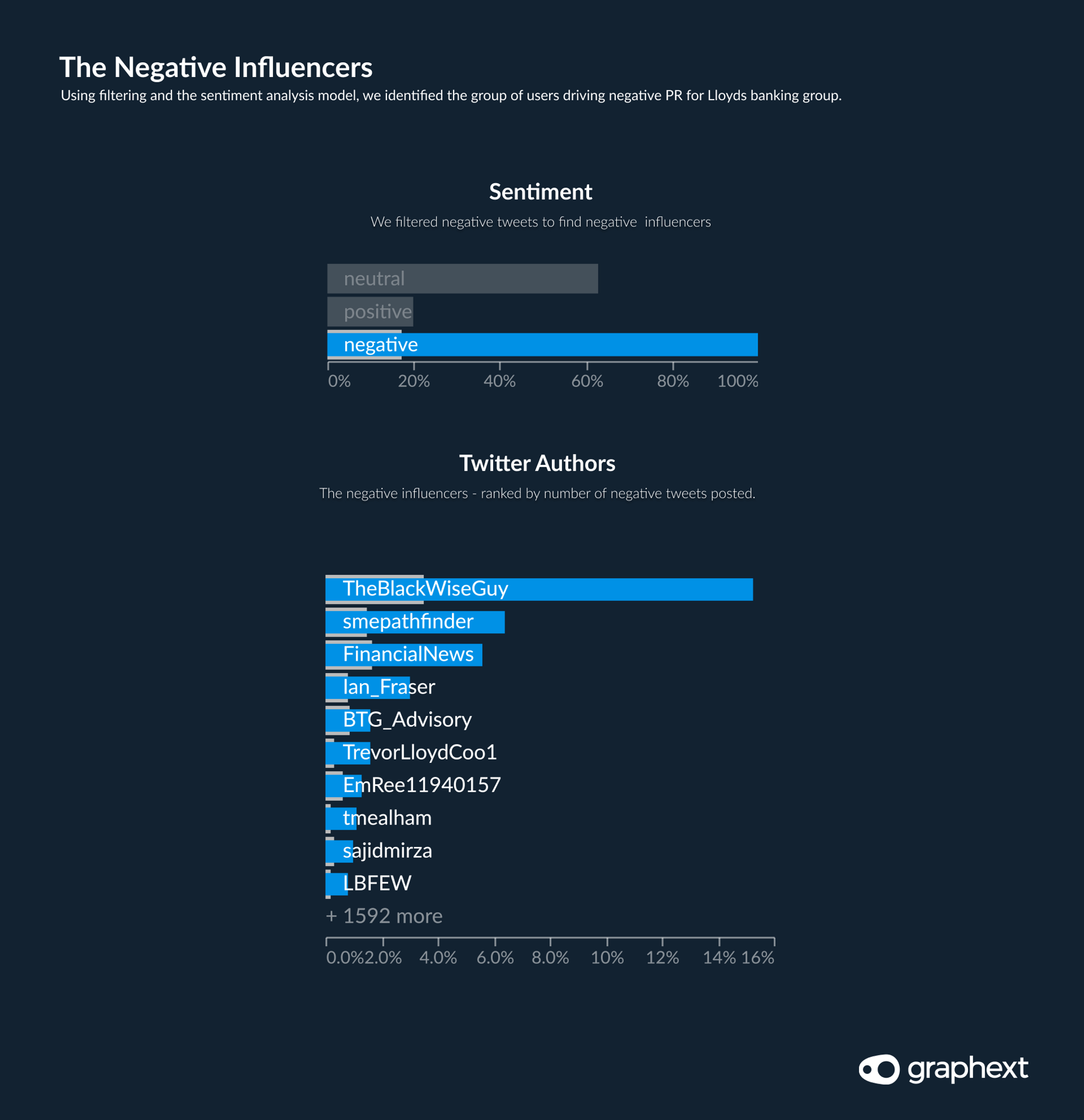
Variable Charts: The negative influencers driving bad PR for Lloyds Banking Group.
Using the results of the Hugging Face sentiment analysis model, we can isolate all negative tweets inside the Graph and decipher who the main influencers are. In this social media analysis project, we can see that the main negative influencers are three specific users; @TheBlackWiseGuy with 15.29% of the negative tweets, @smepathfinder (6.37%) and @FinancialNews at (5.57%). Lloyd's marketing & PR team would then be able to use the topic analysis generated by Graphext to find out what these users were posting negatively about and react accordingly.
This is only scraping the surface of the potential use cases for a topic analysis of Twitter data. Businesses can follow the same analysis structure to perform a competitor analysis, comparing the volume of mentions between your company and your competitors or determining which business has more positive sentiment in tweets talking about them. What's more, marketing teams can use the insights from text analysis of Twitter data to make smart decisions to increase the rate of social media engagement by tracking hashtags and ensuring that some posts contain references to context-specific trending topics for that week. Understanding twitter metrics can truly benefit a social media management team and increase the reach of your company.
On this page
- Scraping Data from Twitter
- Setting Intentions for a Text Analysis of Twitter Data
- Analyzing Conversations on Twitter: A Cluster of Tweets about Competitors & Subsidiary Groups
- Analyzing Conversations on Twitter: Reactions to Business News
- Analyzing Conversations on Twitter: How People Feel About Job Cuts
- Analyzing Conversations on Twitter: Sentiment Analysis
- Analyzing Conversations on Twitter: Finding Influencers
On this page
- Scraping Data from Twitter
- Setting Intentions for a Text Analysis of Twitter Data
- Analyzing Conversations on Twitter: A Cluster of Tweets about Competitors & Subsidiary Groups
- Analyzing Conversations on Twitter: Reactions to Business News
- Analyzing Conversations on Twitter: How People Feel About Job Cuts
- Analyzing Conversations on Twitter: Sentiment Analysis
- Analyzing Conversations on Twitter: Finding Influencers